We build generative AI systems using the most advanced models and frameworks available—GPT-4, Claude, Mistral, LLaMA 2, and other cutting-edge architectures. Our team actively monitors the evolving AI landscape, ensuring your solution leverages the latest trends in transformer-based models, retrieval-augmented generation (RAG), low-rank fine-tuning (LoRA/QLoRA), and token-efficient inference strategies. This keeps your business competitive, performant, scalable, and future-ready.
Generative AI development services
Enhance decision-making, streamline operations, and adapt effortlessly to fast-changing market conditions with state-of-the-art Generative AI systems
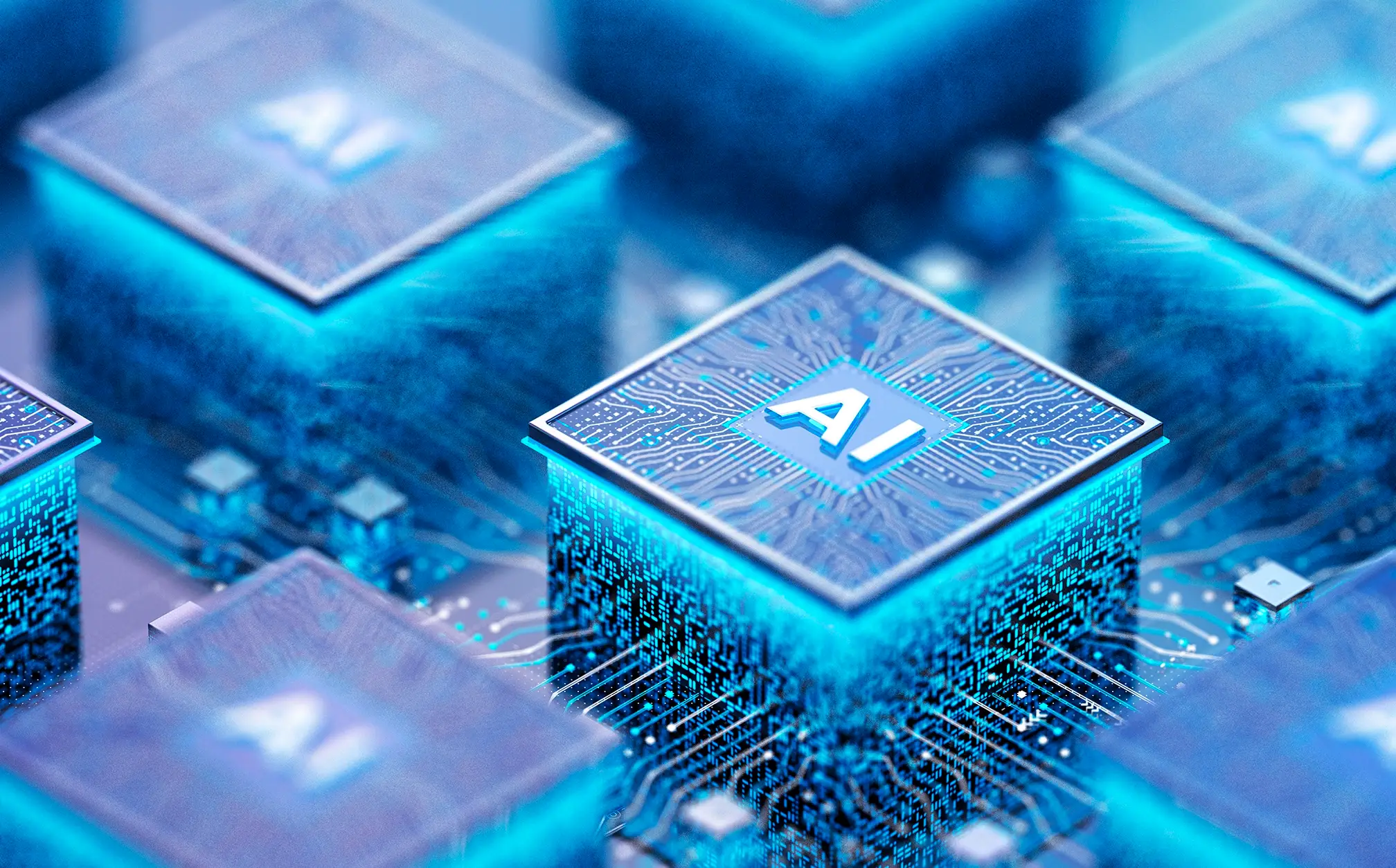
What we offer
We are here to deliver end-to-end generative AI solutions tailored to your industry, infrastructure, and strategic roadmap. Our portfolio includes AI-powered DevOps assistants, knowledge automation tools, intelligent chatbots, and custom LLM-based agents that support decision-making in highly regulated sectors. We have successfully integrated generative AI into both greenfield and legacy systems, ensuring scalability, security, and compliance.
Our offerings cover the entire development lifecycle:
– Ideation and rapid prototyping with tools like Flowise and n8n.
– Data strategy and knowledge pipeline implementation.
– Fine-tuning and deployment of open-source or commercial LLMs.
– Cloud-native and on-premise deployment (AWS, Azure, GCP, Kubernetes).
– Ongoing model evaluation, monitoring, and cost optimization.
Whether you’re considering AI to enhance existing workflows or pioneer new AI-driven capabilities, we bring the engineering expertise and domain knowledge to create a solution not just technically sound—it brings measurable value and is built for long-term profitability.
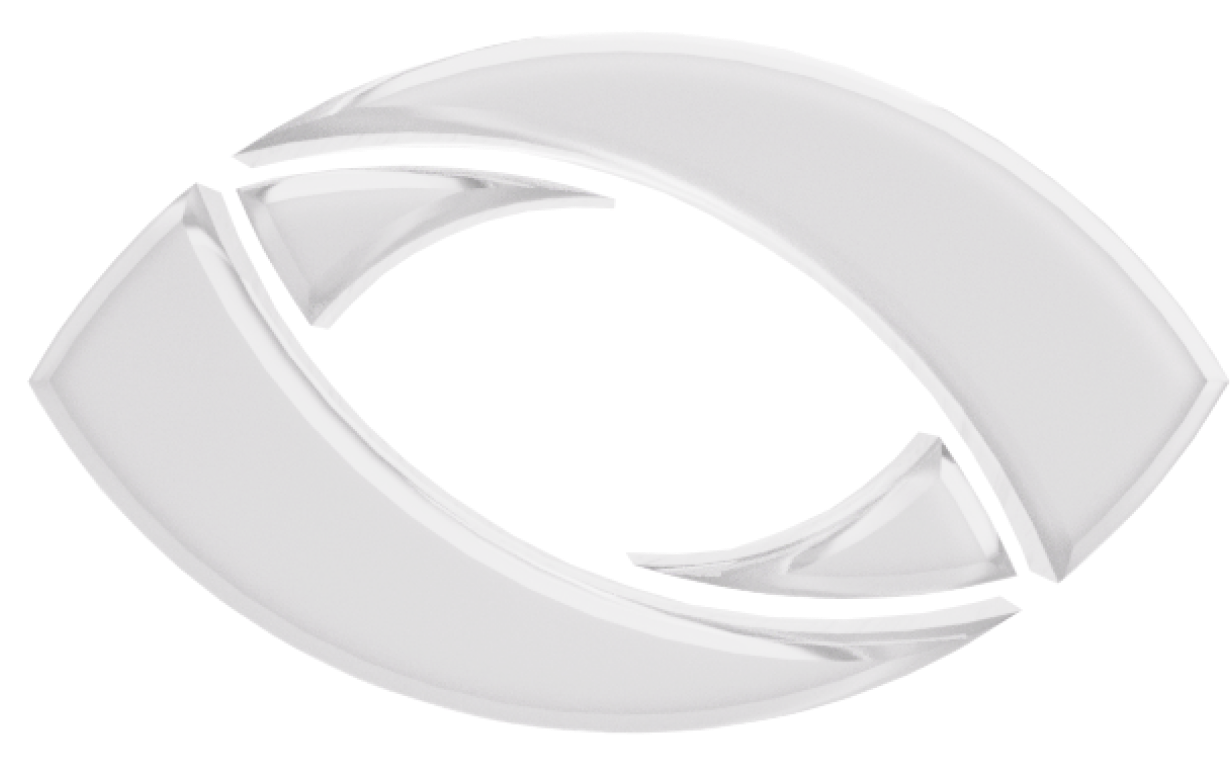
Want to improve your Generative AI services?
Our team is ready to uplevel your Generative AI performance, functionality, and usability.
Our generative AI development services
-
Generative AI strategy and consulting
With our consulting services, we help you define your strategy, evaluate feasibility, and identify high-impact use cases. We assess data readiness and support you in data-related challenges—whether that means consolidating fragmented sources, filling critical gaps, or improving data quality. We select the most suitable models (e.g., GPT, Claude, Mistral, LLaMA) and design scalable solution architectures. Our team guides you through every technical and business decision, from compliance considerations to cost optimisation.
-
Generative AI development services
We build custom AI systems tailored to your use case—from content generation and summarisation to intelligent assistants and retrieval-augmented generation (RAG). Our team handles model orchestration, backend logic, data pipelines, and infrastructure deployment. Whether hosted via commercial APIs or fine-tuned on private infrastructure, we deliver secure, high-performance solutions ready for production.
-
Data engineering for generative AI
We design and implement data pipelines optimised for generative model training, evaluation, and inference. This process includes structured data collection, transformation, embedding, and storage using tools like Supabase, PostgreSQL, and vector databases (e.g., Pinecone, Qdrant). For RAG systems, we ensure efficient chunking, indexing, and semantic search performance across large knowledge bases.
-
Solutions customized to your business
We work with an understanding of each business’ uniqueness. Therefore, we tailor model choice, prompt strategies, infrastructure, and workflows to your specific domain and goals. Whether you’re in healthcare, legaltech, biotech, or SaaS, we develop systems that seamlessly integrate into your environment, deliver business-relevant results, and remain adaptable as your needs evolve.
-
Advanced AI solutions to solve complex problems
For clients with complex requirements, we offer advanced generative AI capabilities, including multi-agent orchestration, fine-tuned LLMs, low-rank adaptation (LoRA), hybrid cloud deployments, and secure on-premise solutions. We also build systems with embedded governance tools, red-teaming protocols, and safety layers to meet the highest standards for enterprise and regulated sectors.
-
Turning prototypes into production-ready AI systems
We take your idea from a quick proof-of-concept to a reliable, scalable, and monitored production system. Starting with no/low-code tools (like Flowise or n8n) for early validation, we then implement backend services, prompt logic, vector storage, and inference APIs to ensure seamless handover to production—with CI/CD and observability built-in.
-
Generative AI integration for businesses
We integrate generative AI into your existing products, platforms, and internal tools. This might include embedding LLMs into SaaS workflows, building agentic RAG systems on top of your document repositories, or enabling AI-driven customer support—all designed for secure access control, scalable API integration, and frictionless deployment. We ensure every integration is timely, stable, and non-disruptive to your core operations.
-
Automating business workflows with AI
We develop AI agents that automate repetitive, time-consuming tasks across departments—marketing, operations, HR, customer service, and more. Using LLMs and orchestration tools, we build systems that execute multi-step processes, route data, respond to queries, and trigger actions across tools—improving speed, accuracy, and resource allocation, and serving as reliable and efficient AI assistants.
-
Empowering smart devices with generative AI
We extend generative AI capabilities to edge and embedded environments, enabling intelligent features in smart devices. We design AI solutions that run efficiently within device constraints, from voice-enabled assistants and on-device summarisation to offline LLM inference using quantised models— all for supporting real-time interaction, automation, and low-latency intelligence at the edge.
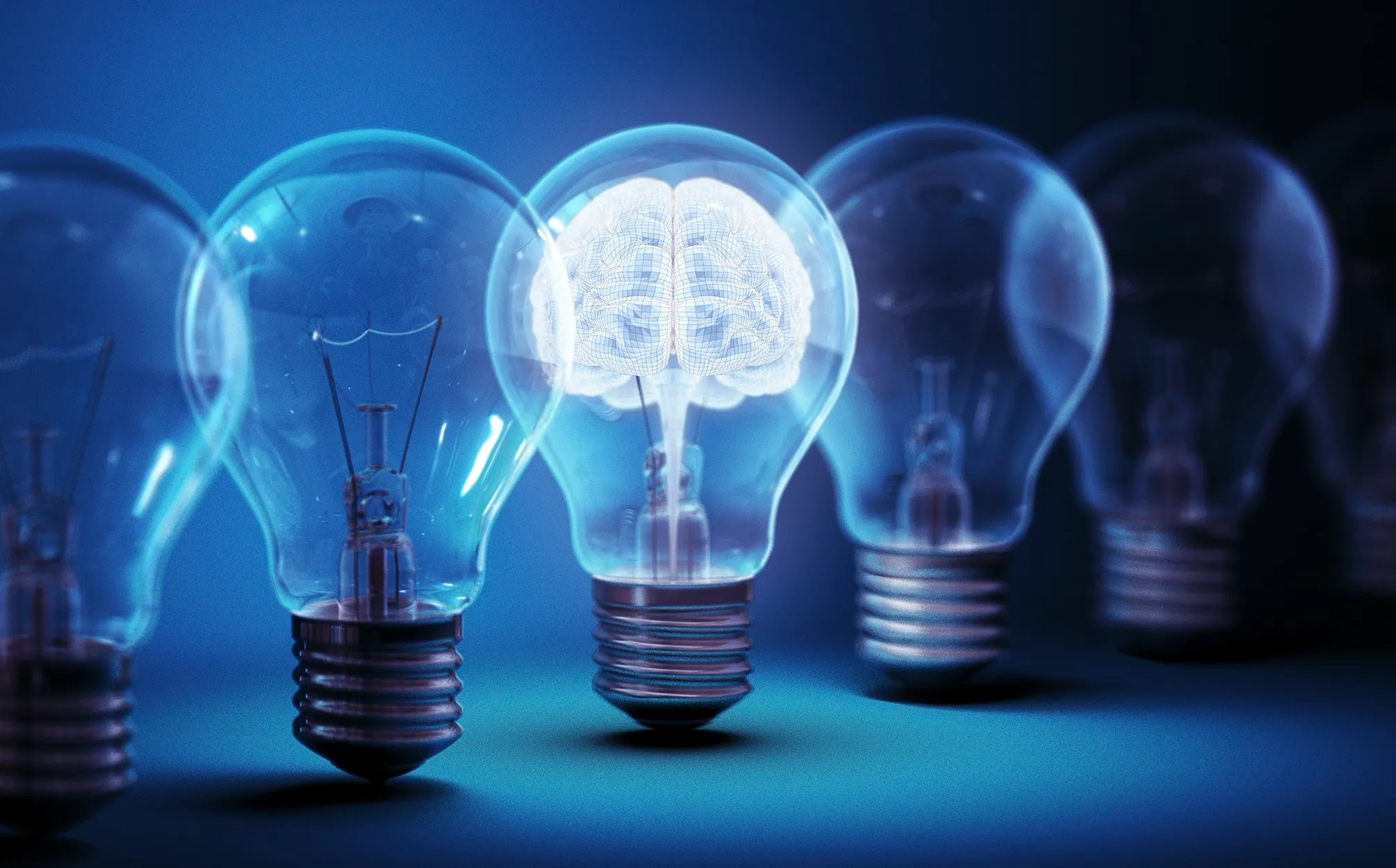
Benefits of generative AI development services
-
State-of-the-art technologies
-
Customised solutions for your specific needs
As it’s been said, we don’t believe in one-size-fits-all AI. Every solution is engineered around your unique data, workflows, and performance requirements. Whether we are talking about a domain-specific chatbot, a legal summarization engine, or an internal document assistant—we adapt model selection, prompt design, data strategy, and deployment architecture to your specific use case. The result is a system that delivers measurable value—not just generic output.
-
A seamless, end-to-end development process
Blackthorn Vision’s full-cycle team includes AI/ML engineers, backend developers, data pipeline architects, DevOps, and product managers. We manage every layer of development—from ideation and prototyping to deployment, monitoring, and post-launch tuning. Such an integrated approach eliminates hand-off delays and quality gaps, bringing consistent progress and allowing for quick pivots and production-grade delivery without friction.
-
Solutions for evolving business needs
As your business grows—whether through higher data volume, increased concurrent users, or feature expansion—our architecture adapts without compromise. We leverage containerized microservices, stateless APIs, and horizontal scaling via orchestration platforms like Kubernetes to ensure consistent performance and no disruptions. We design for flexibility and build scalability into every layer of the systems so that they scale seamlessly across cloud-native, hybrid, or on-premise infrastructures.
-
Optimised costs through intelligent AI automation
We confidently balance both performance and cost-efficiency. By automating repetitive workflows and enhancing decision-making through intelligent generation, we reduce dependency on manual input. We implement token-aware prompting, batch inference, selective model routing (e.g., switching between local and commercial APIs), and smart caching to minimize compute costs. This strategic resource optimization lowers operational expenses while maximizing system impact and long-term ROI.
-
Empowered creativity and productivity
Generative AI incredibly enhances teams’ productivity by streamlining and accelerating content generation and research and ideation processes across roles and departments. This applies to generating marketing copy and technical documentation as well as answering domain-specific queries or designing UI components. By offloading repetitive, low-value tasks, teams can shift their focus toward strategic initiatives that require human attention, significantly accelerating innovation cycles and introducing new creative potential.
Generative AI development process
Generative AI tech stack
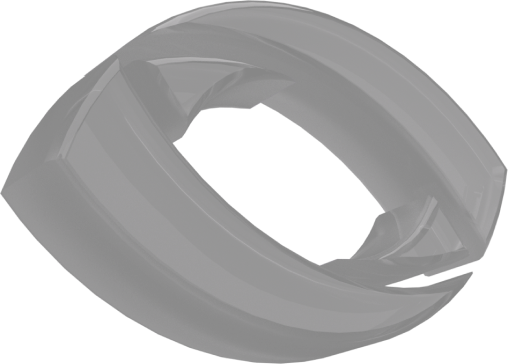
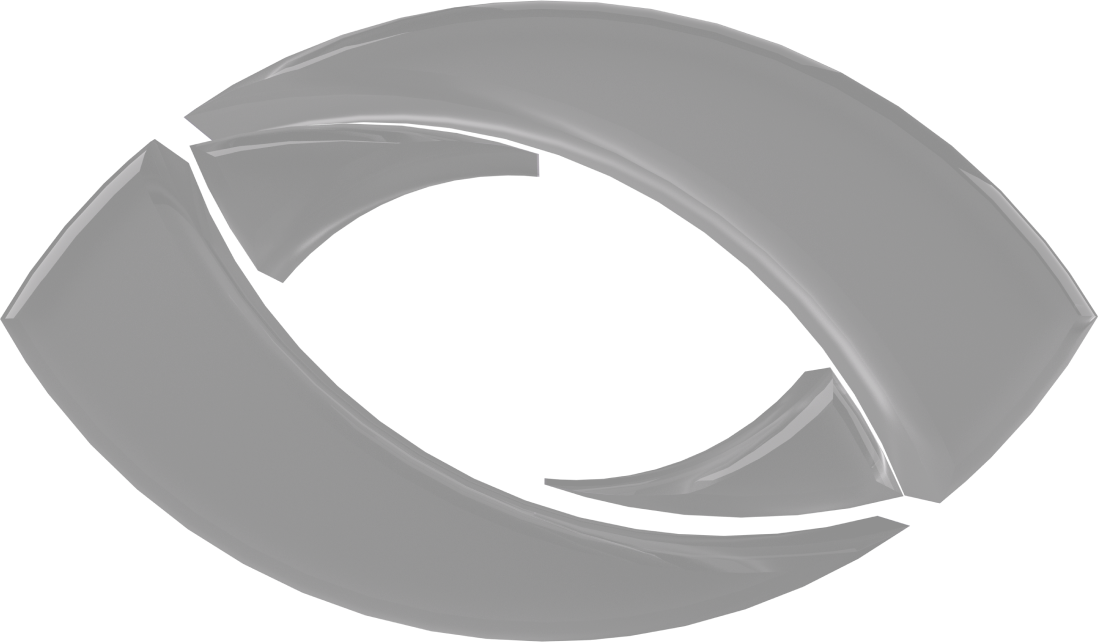
Need to develop your generative AI ?
If you’re ready to transform how your business creates value, we are here to help make it real with our generative AI development services.
Daryna Chorna
Customer success manager
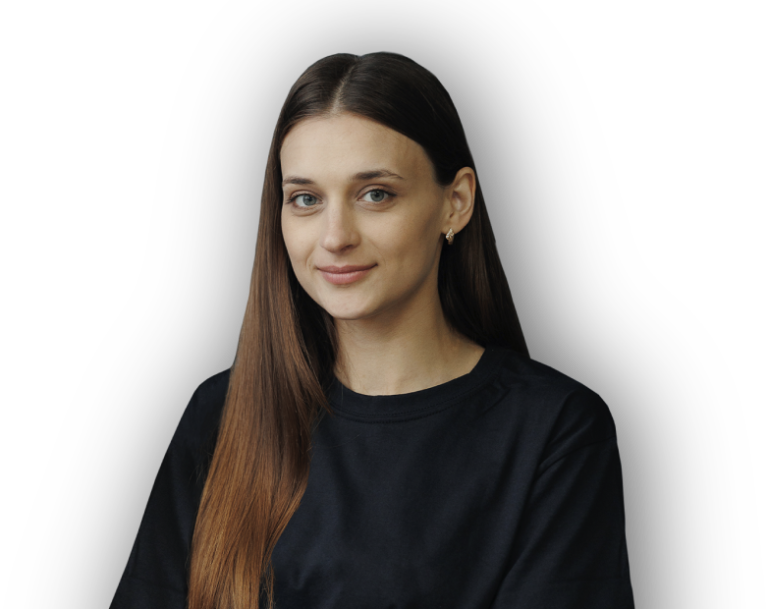
What you achieve with our generative AI development services
What our clients say
4.8
What makes us a great choice for generative AI development?
-
01
Up-to-date, expert engineering
Our cross-functional teams combine deep expertise in AI/ML, backend engineering, DevOps, and secure architecture design. We handle the entire AI lifecycle—from prompt engineering and fine-tuning to full-scale deployment with RAG, vector databases, and inference optimization. Our engineers build scalable, production-grade systems using best practices in API design, CI/CD, and container orchestration. We emphasize modularity, observability, and maintainability to ensure your generative AI product can evolve with your business.
01 /04 -
02
Adaptive tech stack expertise
We work with both proprietary and open-source technologies to meet performance, privacy, and cost requirements.
– LLMs & APIs: OpenAI API, Claude, Mistral, LLaMA, Mixtral, Hugging Face API and models
– RAG & Orchestration: LangChain, LangGraph, LlamaIndex, Haystack
– Embeddings: SentenceTransformers
– Vector Databases: Pinecone, Weaviate, Qdrant
– Storage & Backends: Supabase, PostgreSQL, Firebase, Redis
– Infrastructure: Docker, Kubernetes, AWS/GCP/Azure, Terraform
– Monitoring & Evaluation: Langfuse, Langsmith, OpenAI Evals, Prometheus, Grafana
– Prototyping tools: Flowise, n8n, VoiceflowThis flexible, production-ready stack allows us to move quickly from idea to deployment while maintaining the highest level of performance and reliability. We adapt to your needs and requirements and remain creative to offer the best possible solution for your idea or problem.
02 /04 -
03
Agile development process
We follow an agile, test-driven development model tailored for generative systems. Prototyping begins with quick validation using no/low-code tools, followed by structured data pipelines and modular backend design. Models are tested in isolated stages—prompt tuning, RAG integration, and model behaviour refinement—before full deployment. Each iteration is informed by usage analytics, human feedback, and safety evaluations. Thanks to this approach, we ensure the solution remains aligned with business goals, end-user needs, and model governance standards throughout the lifecycle.
03 /04 -
04
Sector-specific experience
Our generative AI experience spans healthcare, legal-tech, marketing, customer service, and other sectors. We’ve built AI copilots, search agents, content generators, questionnaires, and internal knowledge tools—always aligned with domain-specific compliance, privacy, and performance requirements. This cross-sector exposure allows us to bring tested strategies, reusable components, and proven design patterns to every engagement, reducing both technical and business risk.
04 /04
Generative AI development services: FAQ
-
What is generative AI, and how does it work?
Generative AI refers to machine learning models—such as large language models (LLMs) and generative adversarial networks (GANs)—that create content based on learned patterns in training data. These models produce human-like text, images, audio, or code by predicting and generating sequences or structures. The application range is nearly endless—from healthcare diagnostics and legal summarization to marketing content and game design.
-
What types of projects can benefit from generative AI development services?
Projects across domains can benefit from generative AI, including intelligent customer support, personalised marketing, dynamic content creation, data synthesis for training other AI models, drug discovery, and digital design. Its value lies in automating creativity, improving efficiency, and unlocking new product capabilities that were previously impractical or time-consuming.
-
How can generative AI enhance my business operations or products?
Generative AI can improve your business by automating repetitive creative tasks, generating content, enhancing decision-making through synthetic data and simulations, and accelerating research and development. From daily content marketing to complex industrial design, it supports innovation and reduces time-to-market and operational costs.
-
What is the process for developing a custom generative AI solution?
We follow a structured, collaborative development cycle that includes these steps:
– Discovery and scoping – understand your objectives and constraints
– Data preparation – collect, label, and clean data
– Model selection and prototyping – select the right architecture for your use case
– Development and fine-tuning – train the model and iterate based on feedback
– Testing and evaluation – ensure performance, safety, and bias mitigation
– Deployment and integration – embed into your environment with full scalability
– Ongoing monitoring – track performance and improve over time -
How long does it typically take to develop a generative AI model?
Timelines vary by use case and complexity. A simple proof of concept can take a couple of weeks, while robust, production-grade systems may require several months or more. Key factors include data availability, model architecture, regulatory requirements, and integration needs.
-
What kind of data is needed to train a generative AI model, and how is it handled?
High-quality, domain-specific data is essential. This can include text, images, audio, or structured records depending on the task. We follow best practices for data anonymization, security, and compliance with GDPR, HIPAA, or other relevant standards. Our pipelines include preprocessing, augmentation, and validation to ensure data integrity and model fairness.
-
What support and maintenance services do you offer after the deployment of the AI solution?
We provide post-launch maintenance that includes performance monitoring, system updates, model retraining, and user support. Our team ensures your AI solution stays aligned with evolving business needs and industry changes, as well as data environments, and compliance obligations, keeping it scalable and secure.
Client success stories
Contact us
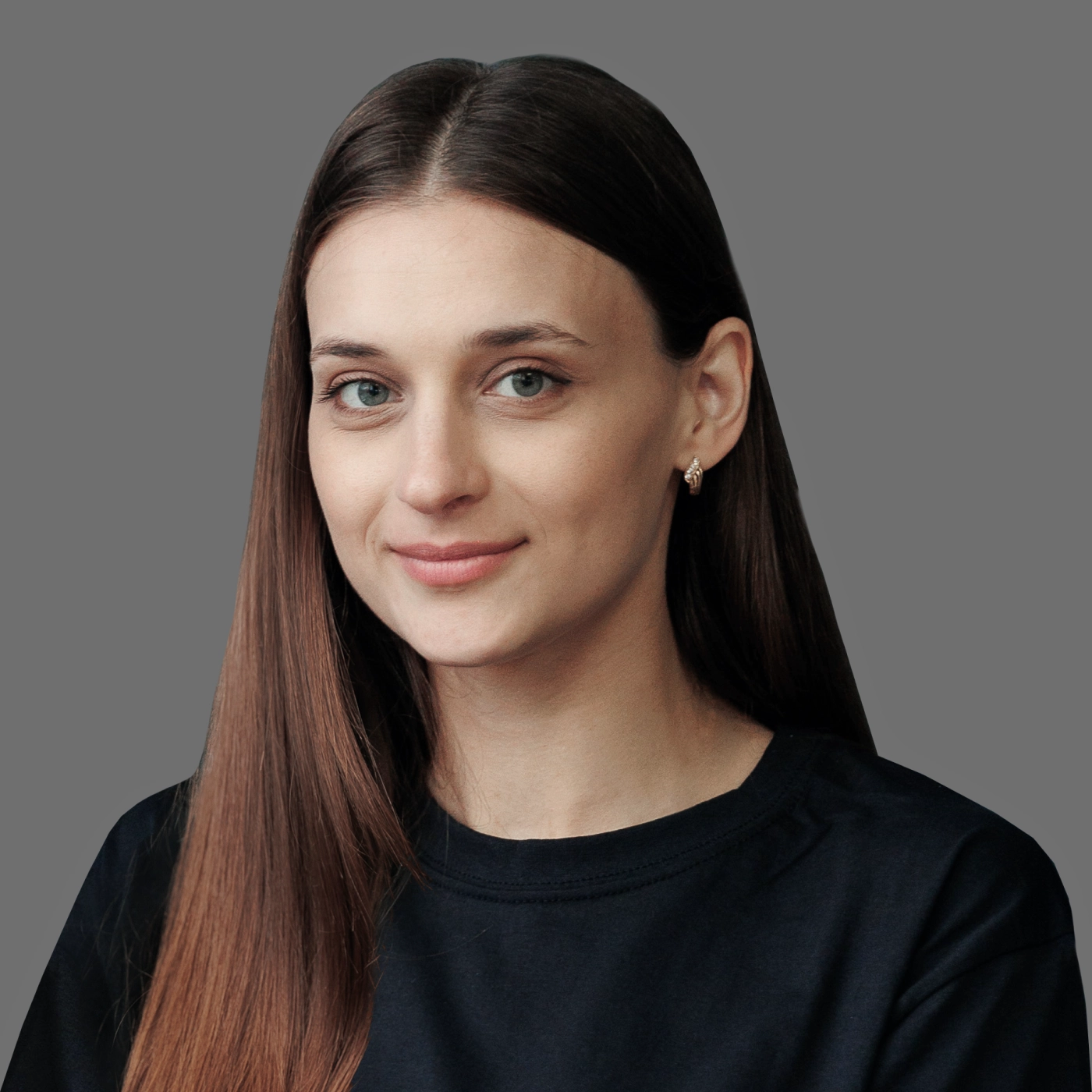
Daryna Chorna
Customer success manager