We use cookies to ensure we give you the best experience on our website. By clicking Agree you accept our Privacy and Cookies Policy.
Machine Learning (ML) in healthcare
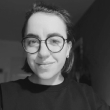
Iryna Hnatiuk
Author
February 27, 2024
Date
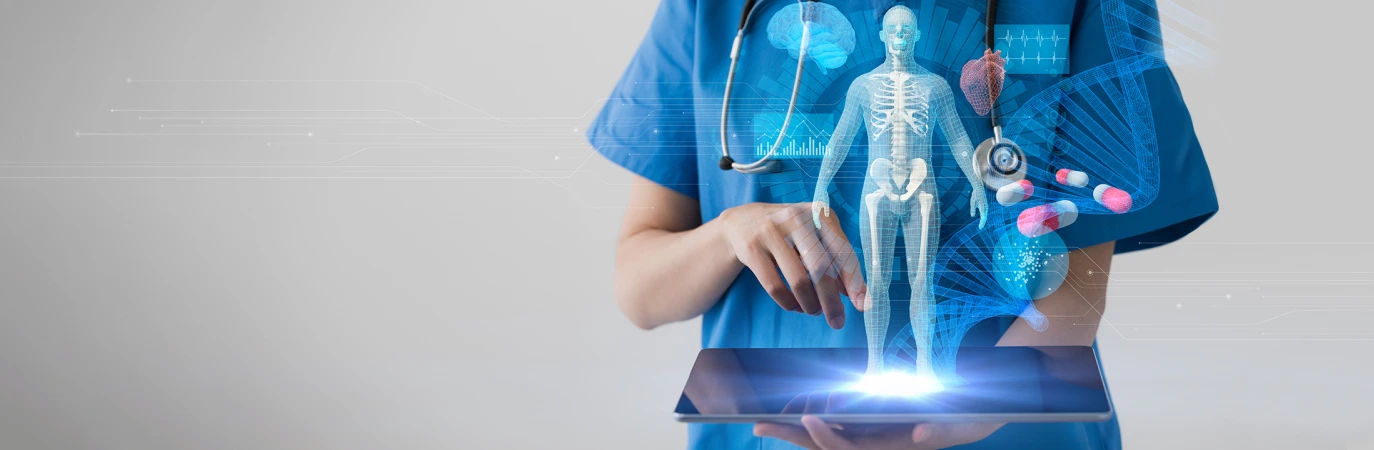
8 minutes read
Content
Healthcare is one of the most data-rich industries in the world, and harnessing this data is critical to improving patient care. Machine Learning is a powerful tool for understanding this data and extracting actionable insights.
Named one of the biggest trends in healthcare in 2023, AI and Machine Learning become more relevant day after day. Soon, we won’t be able to imagine healthcare without these technologies, as their impact on the industry will only continue to grow.
In this article, we’ll explain what Machine Learning is and how it works, explore real-world applications of Machine Learning in healthcare, and discuss potential future uses.
What is Machine Learning?
Machine Learning (ML) is a form of Artificial Intelligence (Al) that lets systems learn from data, identify patterns, and make predictions. The process of Machine Learning is similar to data mining. Both methods search through data and look for patterns. But ML goes a step further – it identifies the data structure, making learning and predictions possible.
There are three types of Machine Learning: supervised learning, unsupervised learning, and reinforcement learning.
Supervised learning involves training a model on labeled data to make predictions about unseen data. An example of supervised learning is a spam filter – once it’s trained on a dataset of emails tagged as spam, it can predict whether future emails are spam or not.
Unsupervised learning involves finding patterns in data without any labeled data. An example of unsupervised learning is clustering – grouping similar data points together.
Reinforcement learning involves an agent making an environment to maximize a reward signal. The agent continually learns from interactions with the environment to improve its decision-making over time.
In summary, ML allows systems to automatically improve their performance on a specific task by learning from data without being explicitly programmed. This helps organizations make better decisions, improve operations, and automate tasks previously performed by humans.
Let’s look at how Machine Learning made its way into healthcare and why it’s here to stay.
Machine Learning in healthcare: a market overview
COVID-19 was a game-changer for Machine Learning in healthcare. The pandemic put immense pressure on the already overloaded healthcare system. Hospitals struggled to meet the needs of patient influx while caring for those with chronic conditions. In addition, the shortage of personal protective equipment (PPE) puts medical personnel’s safety at risk. In response to these challenges, many hospitals have turned to AI-powered solutions.
56% of organizations accelerated their AI deployment after the pandemic. Healthcare providers started to invest more in AI and Machine Learning, with more than half (83%) having an AI strategy in place. As a result, the global AI healthcare market reached$11.6 billion in 2021 and will grow to $187.95 billion by 2030.

4 reasons why Machine Learning is shaping healthcare
There are several factors besides the global pandemic contributing to the growth of Machine Learning in healthcare.
First, it’s the increasing amount of data generated in the healthcare sector: electronic health records (EHRs), medical imaging, genomics data, and more. This data can be used to train ML algorithms to make predictions and uplevel medical services. Additionally, advanced ML techniques like deep learning make it possible to process and analyze large amounts of data to achieve greater accuracy in diagnoses.
The second factor is the growing demand for personalized healthcare services. ML algorithms analyze patient data to identify customized treatment plans, leading to better patient care and disease prevention. On top of that, AI and Machine Learning improve the efficiency of clinical operations, reducing costs and wait times and eliminating human-prone errors.
Another factor contributing to the growth of Machine Learning in healthcare is the amplifying amount of investment going into ML research for healthcare applications. For example, companies like Google have invested in this area over the past years, using AI in genomic analysis, predicting kidney diseases, and various health studies.
Finally, the increasing availability of cloud computing services empowers faster development cycles with Machine Learning projects and scalability to accommodate large datasets. This helps to accelerate the adoption of ML-based solutions across different areas within healthcare.
The future of AI and Machine Learning in the medical field looks promising. Major tech companies have entered the market, and others will follow suit. With the right approach to development, AI and ML will transform the healthcare system, making it more effective and affordable.
But before investing in Machine Learning development, you need to understand how to apply ML algorithms in various medical fields.
But before investing in Machine Learning development, you need to understand how to apply ML algorithms in various medical fields.
Machine Learning applications in healthcare
Machine Learning in healthcare is still in its early stages, but its applications are numerous and varied. Better-informed decisions, higher quality of care, operational efficiencies, and clinical research support are just some of the benefits of ML for healthcare.
Let’s dive deeper into the most popular use cases and real-life examples.
Diagnostic support
The most promising use cases of Machine Learning in healthcare lie within clinical diagnostics. ML algorithms develop predictive models that identify individuals at risk for certain diseases, discover early signs of illness, and recommend treatment options. These models are trained to analyze blood samples, medical images, and other screening results to detect patterns and trends. Here are a few examples of what you can achieve with ML algorithms.
Researchers from Stanford University used Machine Learning to screen chest X-rays for evidence of COVID-19 pneumonia. The system was 94.8% to 98.9% accurate at diagnosing infection and helped overwhelmed radiologists prioritize patients who needed further testing or treatment. In another study, an ML algorithm diagnosed skin cancer with 96% accuracy, outperforming dermatologists.
Lab testing
Laboratory diagnostic tests are typically based on detecting biochemical or morphologic changes. These changes are often subtle and difficult to detect, making it hard to produce reliable results. Machine Learning helps bridge the gap and deliver accurate results faster, saving patient lives.
One successful case of using ML in healthcare is our client’s product. This laboratory diagnostics software utilizes a self-learning algorithm to predict antibiotics’ minimum inhibitory concentration (MIC) given bacterial resistance. Moreover, this ML algorithm assists lab technicians throughout antibiotic susceptibility testing (AST) and ensures error-free results. As a result, patients receive their AST results within 5 hours instead of 24 hours.
Drug discovery
Two common use cases of Machine Learning in healthcare in drug discovery and development include virtual screening and lead optimization. Let’s look at each of them.
Virtual screening identifies new drug targets to treat cancer, Alzheimer’s, and other diseases. The process boils down to this: a library of potential drugs is screened for activity against a particular protein target. However, this method typically requires knowing the 3D structure of the target. Using the deep-learning algorithm that feeds data into neural networks, researchers generate predictions and find new targets for “druggable” proteins.
In lead optimization, ML indicates whether new molecules are more potent or less toxic than existing ones. It solves the common problem of drug development, where most promising drugs fail in clinical trials due to toxicity issues. By using Machine Learning in the medical field to optimize leads early on in drug discovery, scientists reduce the number of failures at later stages.
Personalized care
As patients discharge from the hospital, they fend for themselves in understanding aftercare instructions and managing medications. It’s daunting and overwhelming for those already struggling with their health, and it often leads to re-hospitalization.
For example, nearly 20% of patients with heart failure readmit within 30 days of discharge. Using applications based on Machine Learning in healthcare reduces these numbers by providing support to patients and caregivers during this critical period. ML has the power to solve the issue with personalized recommendations and data-driven insights for faster recovery.
Our team created a smart health tracking app powered by AI and ML algorithms. The platform provides health recommendations based on real-time measurements from wearable devices, lifestyle, genetics, age, and other data. Users receive early notice about dangerous health conditions, personalized workout regimes, nutrition plans, news, and preliminary diagnosis. It continuously improves and adjusts recommendations to come up with even more valuable insights.
In the future, clinicians can use predictive models to identify patients at risk of readmission and provide intensive follow-up care. And you know what the best part is? ML models become more accurate over time. This means that Machine Learning has the potential to reduce hospital readmission rates and improve patient outcomes.

Harness the power of AI and ML with Blackthorn Vision
Healthcare organizations are just beginning to scratch the surface of what’s possible with Machine Learning. As more data is collected and shared, and as ML algorithms become more sophisticated, we will see more successful use cases of Machine Learning in healthcare.
And if you want to build an ML-based solution, it’s vital to partner with a seasoned development team. We tailor our Machine Learning development services to your business goals and unique product vision. Our team will help you gather valuable data and turn this data into insights and predictions that drive tangible results.
At Blackthorn Vision, we have a team of ML and cloud development experts with a proven track record in the healthcare industry. We provide full-cycle software development services for healthcare, including:
- User experience design for medical app
- Cybersecurity in healthcare
- Building custom EHR solutions
- Developing laboratory diagnostics software from scratch
Let’s discuss what we can do for your product! Contact us today and we will offer a solution that matches your needs.